July 12th - 15th, 2024
Virtual Event & In-Person
Drop us a line: info@torontomachinelearning.com
Sponsorships: faraz@torontomachinelearning.com
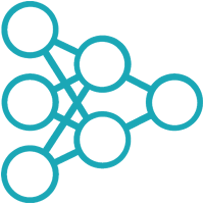
Why Attend
A Unique Experience
For 8 years TMLS has hosted a unique blend of cutting-edge research, hands-on workshops, & vetted industry case studies reviewed by the Committee for your team’s expansion & growth.
We emphasize community, learning, and accessibility.
Join Today
Explore the Uncharted Frontiers of Generative AI
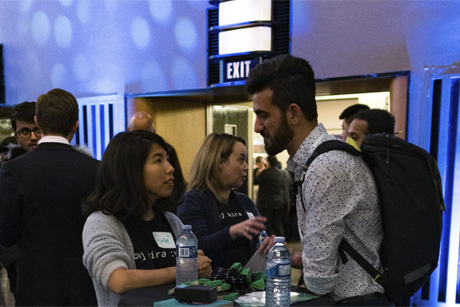
Big Ideas Showcase
See groundbreaking innovations and meet the innovators pushing technological boundaries in Gen-AI.
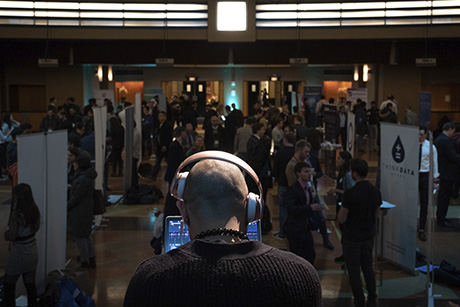
Explore & Network
Event Speakers
See Full Agenda | Reserve your spot today!
Who Attends
2023 Event Demographics
2023 Technical Background
2023 Attendees & Thought Leadership
Business Leaders: C-Level Executives, Project Managers, and Product Owners will get to explore best practices, methodologies, principles, and practices for achieving ROI.
Engineers, Researchers, Data Practitioners: Will get a better understanding of the challenges, solutions, and ideas being offered via breakouts & workshops on Natural Language Processing, Neural Nets, Reinforcement Learning, Generative Adversarial Networks (GANs), Evolution Strategies, AutoML, and more.
Job Seekers: Will have the opportunity to network virtually and meet over 30+ Top Al Companies.
TMLS is a community response addressing the need to unite academic research, industry opportunities and business strategy in an environment that is safe, welcoming and constructive for those working in the fields of ML/AI.
See our team and learn more about the Toronto Machine Learning Society here.
Interested in Partnerships?
Email for Brochure: faraz@torontomachinelearning.com
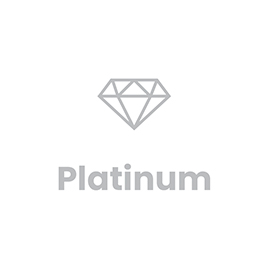
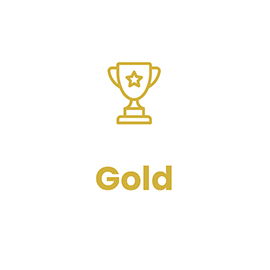
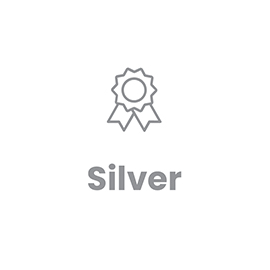
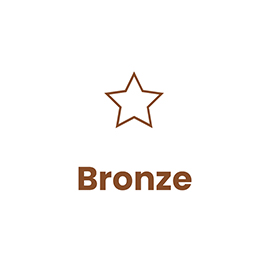